In the fast-evolving world of Artificial Intelligence (AI), researchers and technologists are constantly looking for ways to push the boundaries of computational power and efficiency. One of the most promising avenues for achieving these breakthroughs lies in neuromorphic computing, a revolutionary technology inspired by the human brain. Neuromorphic computing has the potential to reshape the landscape of AI, enabling machines to process information more like humans do, and ultimately paving the way for more intelligent, adaptable, and energy-efficient systems.
But what exactly is neuromorphic computing, and how is it driving AI innovation?
What is Neuromorphic Computing?
Neuromorphic computing refers to the design and development of computing systems that mimic the structure, function, and dynamics of the human brain. The term “neuromorphic” comes from the combination of “neuro” (relating to the brain) and “morphic” (relating to form or structure). These systems aim to emulate biological neural networks, which form the foundation of human intelligence.
Traditional computing relies on a centralized processing model that follows binary logic to execute tasks. In contrast, neuromorphic computing uses spiking neural networks (SNNs), which simulate how neurons fire electrical signals in the brain. This innovative approach offers the potential for faster, more efficient information processing, particularly when it comes to tasks involving pattern recognition, learning, and decision-making.
The neuromorphic computing industry is expected to grow from USD 28.5 million in 2024 and is estimated to reach USD 1,325.2 million by 2030; it is expected to grow at a Compound Annual Growth Rate (CAGR) of 89.7% from 2024 to 2030.
Growth in the neuromorphic computing industry is driven through the integration of neuromorphic computing in automotive and space operations. In space, where bandwidth is limited and the communication delay might be considered large, onboard processing capabilities are crucial. The neuromorphic processor analyzes and filters data at the point of collection, reducing the need to transmit large datasets back to Earth. whereas, in automobile sector, neuromorphic processors can make autonomous driving systems more responsive by onboard real-time processing with minimal latency so that safety is ensured along with efficiency.
Download PDF Brochure @
https://www.marketsandmarkets.com/pdfdownloadNew.asp?id=227703024
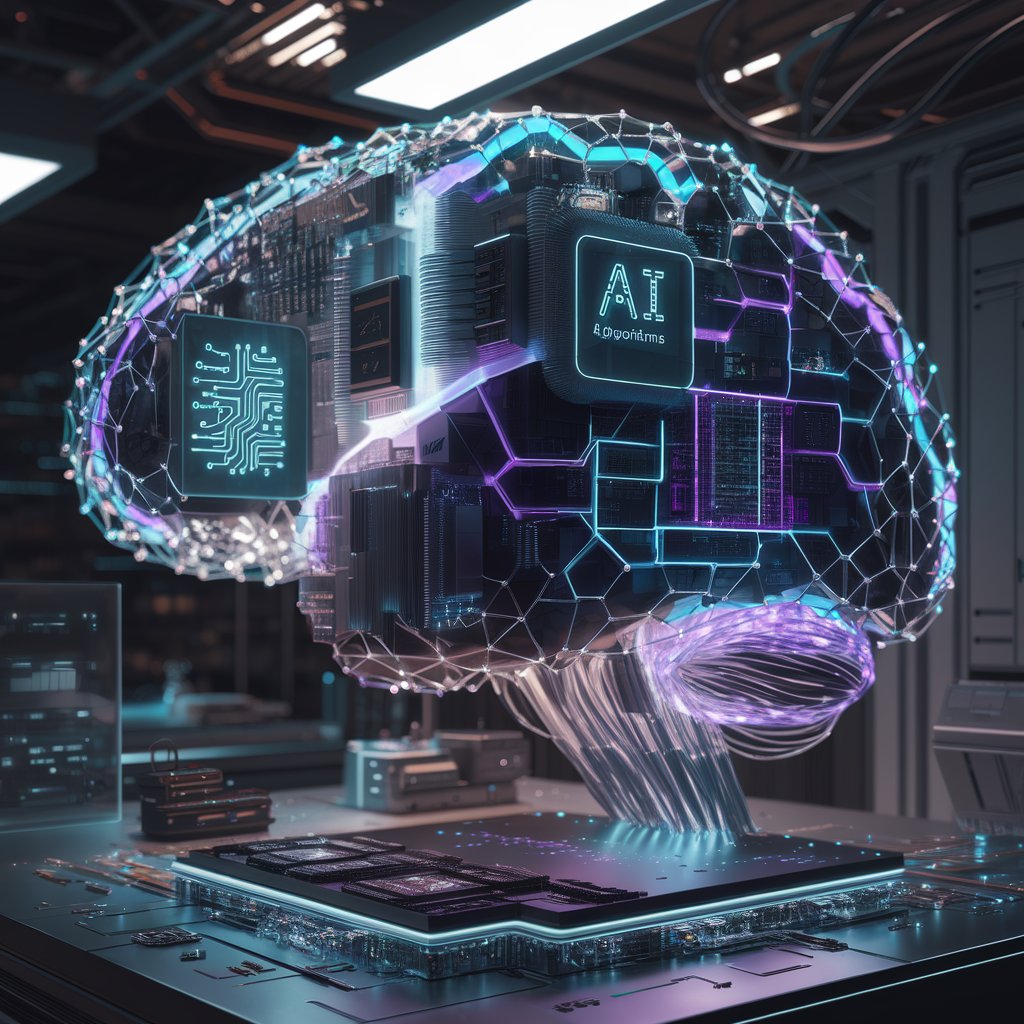
Key Features of Neuromorphic Computing:
-
Brain-Like Processing: Neuromorphic systems use artificial neurons and synapses to process information, much like how the brain processes sensory input. These systems communicate via spikes, representing the electrical signals in neurons, enabling real-time processing and learning.
-
Energy Efficiency: One of the most significant advantages of neuromorphic computing is its low energy consumption. In comparison to traditional computing models that require massive amounts of power, neuromorphic chips are designed to use less energy, mimicking the efficiency of biological systems. This energy efficiency makes neuromorphic computing an attractive option for edge computing devices, such as IoT sensors, autonomous systems, and mobile devices.
-
Adaptability and Learning: Just as the brain learns from experience and adapts to new information, neuromorphic computing systems can learn and adapt without requiring pre-programmed instructions. These systems are capable of unsupervised learning, where they can adjust their behavior based on patterns in the data they process. This dynamic capability enables them to handle real-time changes in environments and tasks, which is essential for AI applications like autonomous vehicles and robotics.
-
Parallel Processing: Neuromorphic computing excels in parallel processing, meaning that many tasks can be performed simultaneously rather than sequentially. This makes neuromorphic systems ideal for handling complex, large-scale tasks, such as natural language processing, image recognition, and predictive analytics.
The Role of Neuromorphic Computing in AI Innovation
The integration of neuromorphic computing into AI systems holds the potential to significantly enhance the capabilities of artificial intelligence across various sectors. Here’s how neuromorphic computing is revolutionizing the future of AI:
1. Enhanced Cognitive Computing
One of the primary goals of neuromorphic computing is to create systems that exhibit cognitive capabilities similar to those of the human brain. Cognitive computing refers to systems that can simulate human thought processes, such as learning, reasoning, and decision-making. By using brain-like structures, neuromorphic systems can develop a deeper understanding of the environment, enabling smarter, more intuitive AI applications.
For example, cognitive AI systems powered by neuromorphic computing can analyze vast amounts of unstructured data, identify patterns, and make predictions or recommendations with a level of sophistication similar to human thinking. This technology has the potential to transform industries such as healthcare, finance, customer service, and more, by providing deeper insights and more accurate decision-making.
2. Faster and Smarter AI Models
Traditional AI models, such as deep learning and neural networks, require significant computational power and time for training and inference. In contrast, neuromorphic computing enables faster, real-time processing by simulating the brain’s ability to process information efficiently. With neuromorphic systems, AI models can be trained and deployed more quickly, leading to faster decision-making and quicker responses to changing conditions.
Moreover, neuromorphic computing enhances machine learning models by enabling unsupervised learning. This allows AI systems to learn from new experiences and adapt to unforeseen challenges without needing extensive labeled datasets. The ability to quickly adapt and learn in real time is essential for applications such as autonomous vehicles and robotics, where unexpected scenarios often occur.
3. Autonomous Systems and Robotics
Neuromorphic computing is a game-changer for autonomous systems and robotics. These systems require rapid, adaptive decision-making in dynamic, real-world environments. Whether it’s an autonomous vehicle navigating through traffic or a robot performing complex tasks in a factory, the ability to process sensory data and adjust actions in real time is critical.
Neuromorphic systems excel in processing sensory information, such as visual, auditory, or tactile data, which is necessary for robot perception. With faster processing times and better learning capabilities, neuromorphic computing can enable robots to make more accurate decisions, improve efficiency, and adapt to new tasks with minimal human intervention.
4. Edge AI and Internet of Things (IoT)
The rise of edge AI and IoT devices has spurred a need for more efficient computing systems capable of processing data locally, rather than relying on cloud servers. Neuromorphic computing fits perfectly into this landscape due to its low power consumption and ability to process data in real time.
Edge devices equipped with neuromorphic chips can perform complex tasks without the need for constant communication with centralized servers, reducing latency and improving system responsiveness. This is particularly important for applications such as smart cities, predictive maintenance, and healthcare monitoring, where quick decision-making is essential.
5. AI for Sustainable Development
Another exciting opportunity presented by neuromorphic computing is its potential to contribute to sustainable development. The energy efficiency of neuromorphic systems aligns well with global efforts to reduce carbon footprints and create environmentally friendly technologies. Neuromorphic computing can play a crucial role in advancing green technologies, such as smart grids, renewable energy systems, and environmental monitoring.
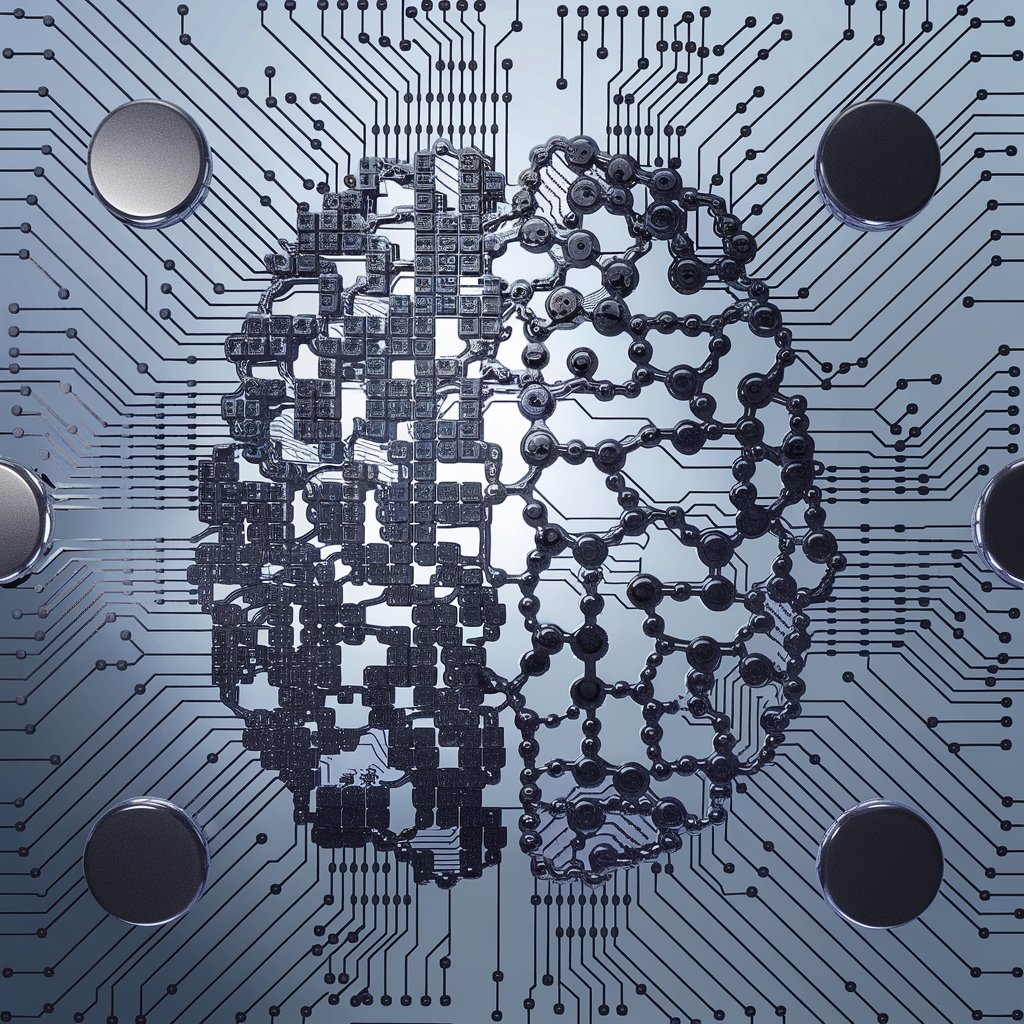
Challenges and Future Outlook
While neuromorphic computing holds immense promise, it is still in the early stages of development. Challenges such as hardware limitations, the need for specialized software frameworks, and the complexity of designing brain-like architectures must be addressed before neuromorphic systems can be widely adopted.
However, as research in this field continues to progress, we can expect significant advancements in neuromorphic hardware, software, and applications. In the near future neuromorphic computing could become a cornerstone of AI, driving innovations across industries and helping us achieve more intelligent, efficient, and sustainable technologies.
Neuromorphic computing is poised to play a pivotal role in the next generation of AI technologies. By mimicking the structure and function of the human brain, neuromorphic systems promise to deliver faster, smarter, and more energy-efficient AI solutions. From cognitive computing and robotics to edge AI and sustainable development, the possibilities are vast. As we continue to unlock the potential of neuromorphic computing, we are one step closer to achieving true artificial intelligence that can think, learn, and adapt just like the human mind. The future of AI is bright, and neuromorphic computing is at the heart of this exciting journey.
frequently asked questions (FAQs) about Neuromorphic Computing:
1. What is neuromorphic computing?
Neuromorphic computing refers to the design of computer systems that mimic the structure and function of the human brain. Using artificial neurons and synapses, neuromorphic systems aim to replicate brain-like processes such as learning, pattern recognition, and decision-making. Unlike traditional computing that relies on binary logic, neuromorphic computing uses spikes and dynamic interactions similar to the way neurons fire in the brain.
2. How is neuromorphic computing different from traditional computing?
Traditional computing systems rely on sequential logic and binary operations (0s and 1s) to process information. In contrast, neuromorphic computing uses spiking neural networks (SNNs) that operate in parallel, mimicking the behavior of biological neural networks. This allows neuromorphic systems to be more efficient in processing complex tasks such as learning and pattern recognition, while consuming less energy.
3. What are the key benefits of neuromorphic computing?
-
Energy Efficiency: Neuromorphic systems are designed to use significantly less power compared to traditional computing models, making them ideal for edge computing and mobile devices.
-
Real-time Learning and Adaptability: Neuromorphic systems can learn on-the-go and adjust their behavior based on new data, similar to how the human brain adapts to new experiences.
-
Enhanced Cognitive Capabilities: By mimicking brain-like processing, neuromorphic systems are better at handling complex, unstructured data and decision-making tasks.
-
Parallel Processing: Neuromorphic systems can perform multiple tasks simultaneously, offering a performance boost for complex AI applications.
4. What industries can benefit from neuromorphic computing?
Neuromorphic computing has applications in several industries, including:
- Autonomous Vehicles: For real-time decision-making and learning from the environment.
- Robotics: For adaptive, brain-like responses in dynamic environments.
- Healthcare: For faster data analysis in diagnostics, medical imaging, and patient monitoring.
- IoT and Edge AI: For reducing latency and power consumption in edge devices.
- Finance and Security: For fraud detection, risk analysis, and anomaly detection.
5. How does neuromorphic computing contribute to AI advancements?
Neuromorphic computing allows AI models to process information more efficiently and quickly, enabling faster and more accurate decision-making. By enabling unsupervised learning, neuromorphic systems do not require extensive labeled datasets, which improves their ability to handle real-world challenges. They enhance AI’s cognitive capabilities, making systems smarter, more adaptive, and more autonomous.
6. Is neuromorphic computing already being used in real-world applications?
While neuromorphic computing is still in the early stages of development, there are several promising applications already emerging. Companies like Intel (with its Loihi chip) and IBM (with TrueNorth) have developed neuromorphic chips that are being used in research for applications such as robotics, edge AI, and healthcare.
7. What are the challenges in developing neuromorphic computing systems?
-
Hardware Development: Designing neuromorphic hardware that accurately mimics the human brain is technically challenging and requires significant resources.
-
Software Integration: Developing software that can effectively interact with neuromorphic systems is still a work in progress. There is a need for specialized programming models to optimize the use of neuromorphic hardware.
-
Scalability: Scaling neuromorphic systems to handle large datasets and real-world tasks remains a challenge.
8. What is the future of neuromorphic computing?
The future of neuromorphic computing is promising, with continued advancements in both hardware and software. As more industries explore the potential of AI, neuromorphic systems will play a pivotal role in pushing the boundaries of intelligent and adaptive systems. The field is expected to impact industries such as healthcare, robotics, autonomous systems, and IoT, driving the development of smarter, energy-efficient solutions for the future.
9. How does neuromorphic computing help in sustainability?
Neuromorphic computing’s energy efficiency makes it an important technology for the future of sustainable AI. By reducing the power consumption needed for computation, neuromorphic systems can support applications that require high performance while minimizing environmental impact. This aligns well with global sustainability efforts to develop eco-friendly, energy-efficient technologies.
10. Can neuromorphic computing replace traditional AI models?
While neuromorphic computing shows great promise, it is unlikely to completely replace traditional AI models in the near future. Instead, it will complement existing AI technologies by offering more specialized solutions for tasks that require brain-like capabilities, such as real-time learning, pattern recognition, and adaptive decision-making. As technology evolves, we may see a hybrid model that combines the strengths of both neuromorphic and traditional computing.