As the backbone of the digital economy, data centers have long depended on powerful chips to handle vast and complex workloads. But with artificial intelligence (AI) workloads growing exponentially—from generative models like GPT to real-time recommendation engines and deep learning inference—traditional chip architectures and data center infrastructures are being reimagined. AI isn’t just reshaping the software stack; it’s fundamentally transforming the data center chip industry itself.
From CPUs to AI Accelerators: The New Silicon Standard for Data Centers
The AI boom has created an inflection point for chip architecture in data centers. CPUs, once the dominant compute engines, are now often paired—or outright replaced—by specialized AI accelerators like GPUs, TPUs, and custom ASICs.
Tech giants like Google, Microsoft, and Amazon are designing their own chips tailored to AI workloads, enabling faster inference, lower latency, and reduced energy consumption. Meanwhile, startups are challenging traditional players with novel chip designs built from the ground up for AI parallelism.
As workloads shift, data centers are retooling themselves around these new chips, with software stacks and network fabrics optimized for AI-first architectures.
AI Is Reinventing Chip Design—Starting With the Data Center
Artificial intelligence isn’t just running on data center chips—it’s now designing them.
AI-driven tools are revolutionizing chip design workflows, enabling automated floorplanning, performance tuning, and power optimization. These advances slash design cycles and reduce human error, while unlocking new performance gains.
Google’s AI-designed TPU chips are an early proof of concept, but the trend is expanding industry-wide. EDA giants like Synopsys and Cadence are integrating AI into their design suites, marking the beginning of fully AI-assisted silicon engineering.
Download PDF Brochure @
https://www.marketsandmarkets.com/pdfdownloadNew.asp?id=39999570
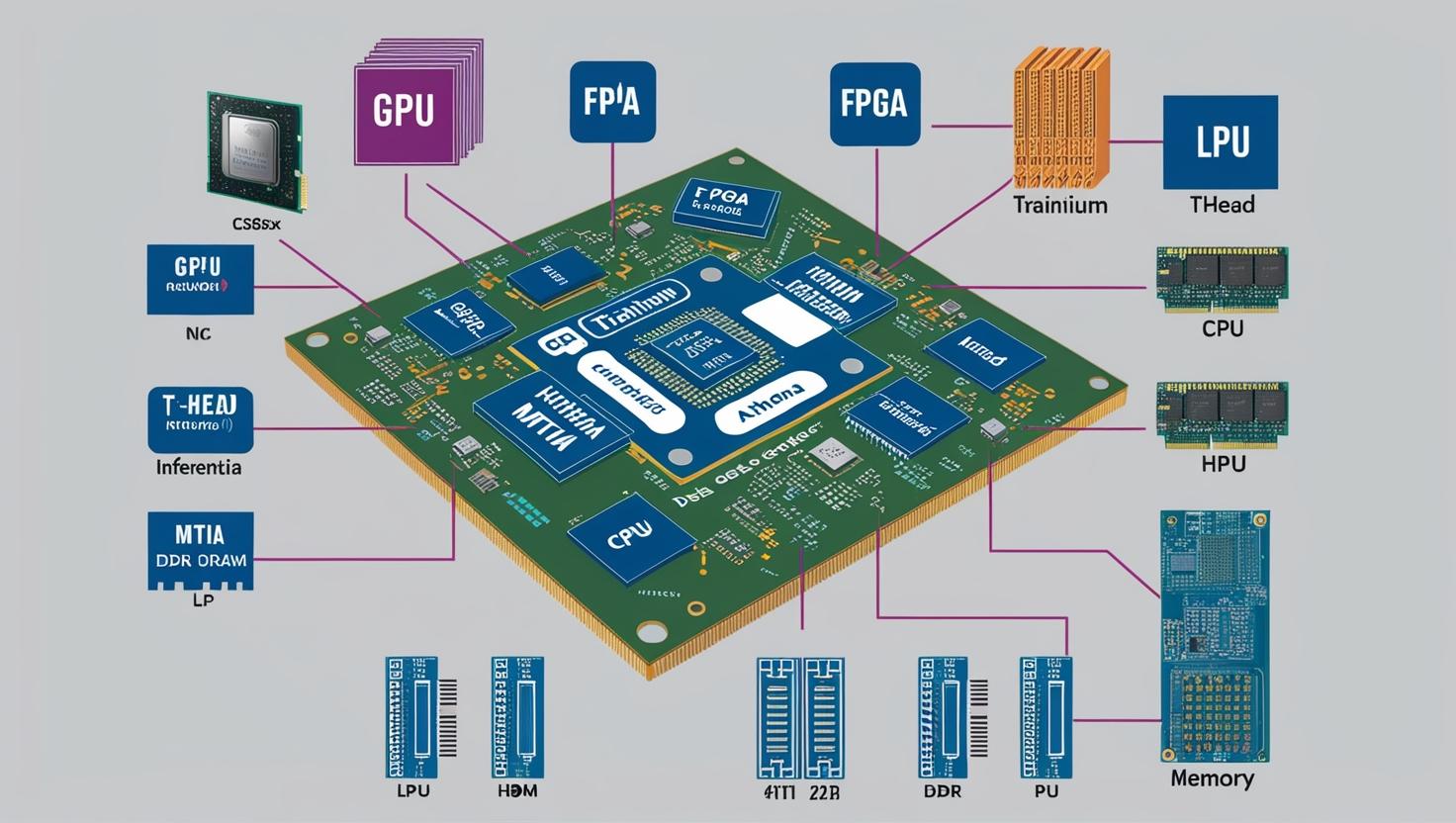
Building AI for AI: The Feedback Loop Fueling Chip Innovation
The relationship between AI and data center chips has become self-reinforcing: AI needs better chips to scale, and AI is now essential to designing those better chips.
This feedback loop is accelerating innovation across the silicon supply chain. From architecture search to thermal simulation and layout optimization, AI is compressing development timelines and creating more efficient, scalable chips tailored for AI inference and training.
This new model of AI-developed, AI-deployed, AI-powered silicon is defining the future of cloud and enterprise compute infrastructure.
Why AI Is Breaking the Traditional Data Center Mold
Traditional data center design is reaching its limits as AI models grow more complex and compute-hungry. Training large language models or deploying real-time AI inference requires tightly integrated systems—from chip to rack.
In response, hyperscalers are embracing heterogeneous architectures, chiplet-based designs, liquid cooling systems, and high-bandwidth memory configurations that support AI chip performance.
New workloads demand new layouts—and AI is both the cause and the architect of this transformation.
The Data Center Arms Race: AI Chips and the Battle for Silicon Dominance
As demand for AI compute explodes, chipmakers and cloud providers are locked in a high-stakes arms race to develop the fastest, most efficient processors.
-
NVIDIA leads with GPU dominance, but rivals like AMD, Intel, and dozens of startups are hot on their heels.
-
Google, Amazon, and Microsoft are investing billions in custom AI chips to gain control over performance, supply chains, and cost.
-
Fab innovation from TSMC and Samsung is critical to meet the transistor density required for AI workloads.
The result is a fragmented yet thriving ecosystem where innovation is accelerating at every layer of the stack.
AI at the Edge, Powered by the Cloud: A New Role for Data Center Chips
While AI inference is moving to edge devices, training remains anchored in powerful cloud data centers. This hybrid architecture demands chips that can not only train massive models but also support fast model updates and edge deployment.
New chip architectures are being optimized to support this cloud-to-edge AI lifecycle, with better memory management, higher interconnect bandwidth, and support for model compression and distillation.
Data center chips are becoming orchestrators of distributed intelligence—not just compute engines.
The Green Imperative: AI Chips and Sustainable Data Center Design
With AI workloads consuming vast amounts of power, energy efficiency is no longer optional. AI chips are at the forefront of a new push toward sustainable data center design.
From low-power inferencing chips to dynamic workload scheduling and liquid cooling, the industry is innovating fast to reduce carbon footprints. AI itself is helping optimize energy consumption, balancing compute performance with sustainability goals.
The green data center of the future will be powered by chips designed not just for speed, but for smart energy use.