The integration of artificial intelligence (AI) into the battery energy storage system (BESS) industry is reshaping the global energy landscape. As the world transitions toward renewable energy and sustainable practices, the AI impact on battery energy storage system industry has become a pivotal force in optimizing energy storage, enhancing battery performance, and driving cost efficiencies.
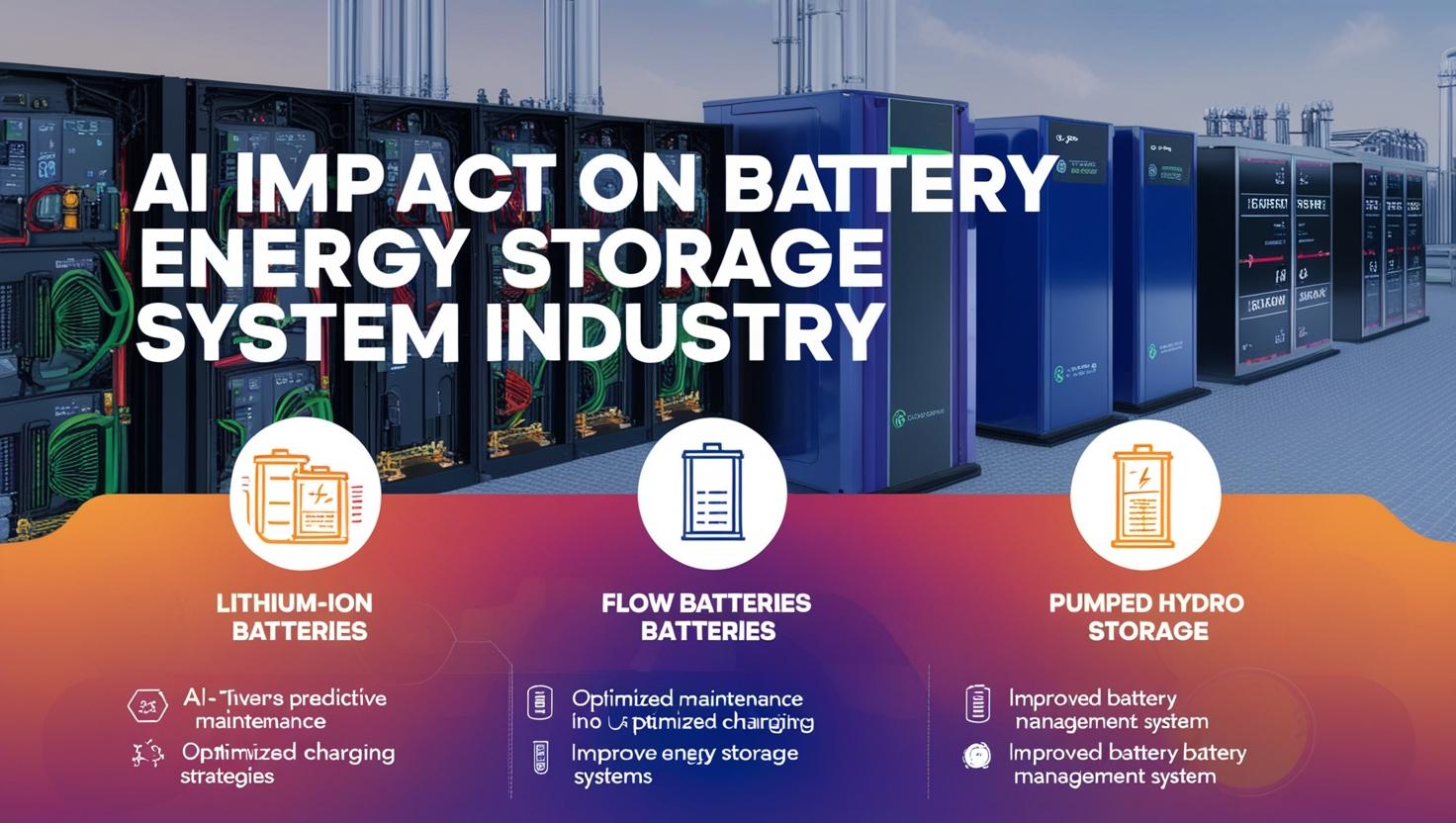
Understanding the Core of Battery Energy Storage Systems and AI’s Transformative Role
Battery energy storage systems are critical components of modern energy infrastructure, enabling the storage of electricity from renewable sources like solar and wind for use during peak demand or low production periods. These systems, often powered by lithium-ion batteries, ensure grid stability, support renewable energy integration, and reduce reliance on fossil fuels. However, traditional BESS operations face challenges such as battery degradation, inefficient energy management, and high maintenance costs. This is where the AI impact on battery energy storage system industry comes into play, addressing these limitations through intelligent automation and data-driven insights.
AI technologies, including machine learning, deep learning, and neural networks, analyze vast amounts of data generated by BESS operations. By processing real-time information on battery health, charge cycles, and environmental conditions, AI optimizes performance and extends battery lifespan. For instance, AI-driven predictive maintenance can identify potential faults before they occur, reducing downtime and maintenance costs. The AI impact on battery energy storage system industry is not just about improving efficiency but also about enabling smarter, more sustainable energy ecosystems that align with global decarbonization goals.
Enhancing Battery Performance Through AI-Driven Optimization Techniques
One of the most significant aspects of the AI impact on battery energy storage system industry is its ability to enhance battery performance. Batteries degrade over time due to repeated charge-discharge cycles, temperature fluctuations, and overuse. Traditional battery management systems (BMS) rely on static algorithms that cannot adapt to dynamic conditions. AI, however, introduces adaptive control systems that continuously learn from battery usage patterns.
Machine learning models analyze parameters such as state-of-charge (SoC), state-of-health (SoH), and temperature to optimize charging and discharging cycles. For example, AI can adjust charging speeds to prevent overcharging, which reduces stress on battery cells and extends lifespan.
Moreover, the AI impact on battery energy storage system industry extends to thermal management. Overheating is a major concern in high-performance BESS applications. AI-driven thermal management systems use real-time data to dynamically adjust cooling mechanisms, ensuring batteries operate within safe temperature ranges. This not only enhances safety but also improves energy efficiency by reducing unnecessary cooling, further solidifying AI’s role in transforming battery performance.
Predictive Maintenance: A Game-Changer for Battery Energy Storage Systems
Maintenance is a significant cost driver in the BESS industry, with unexpected failures leading to downtime and financial losses. The AI impact on battery energy storage system industry is particularly evident in predictive maintenance, which leverages AI algorithms to anticipate and prevent issues before they escalate. By analyzing historical and real-time data, AI can detect anomalies in battery performance, such as irregular voltage drops or capacity fade, and predict when maintenance is required.
For instance, deep learning models can identify patterns associated with battery wear and tear, enabling operators to replace faulty components proactively. This approach has been shown to reduce fire risks by up to 70% in lithium-ion batteries, addressing one of the industry’s biggest safety concerns. Predictive maintenance also minimizes operational disruptions, ensuring continuous power supply for critical applications like data centers and hospitals. The AI impact on battery energy storage system industry thus translates into significant cost savings and improved reliability, making BESS more attractive for widespread adoption.
Optimizing Energy Management for Grid Stability and Renewable Integration
The integration of renewable energy sources into the grid is a cornerstone of the global energy transition, but their intermittent nature poses challenges for grid stability. The AI impact on battery energy storage system industry addresses this by optimizing energy management and enabling seamless integration of renewables. AI algorithms forecast energy demand and supply, determining the optimal times to store or release energy based on real-time grid conditions and pricing.
For example, AI can prioritize storing energy during periods of low demand or high renewable production and releasing it during peak hours to prevent blackouts. This dynamic energy management improves grid efficiency by up to 40%, making renewable energy storage systems more effective. Additionally, AI supports microgrid operations by managing decentralized energy resources, such as rooftop solar panels and small-scale BESS, ensuring local energy self-sufficiency.
The AI impact on battery energy storage system industry also extends to demand response programs, where AI predicts consumption patterns and adjusts BESS operations to balance grid loads. This not only enhances grid resilience but also reduces energy costs for consumers and utilities, reinforcing AI’s transformative potential in the energy sector.
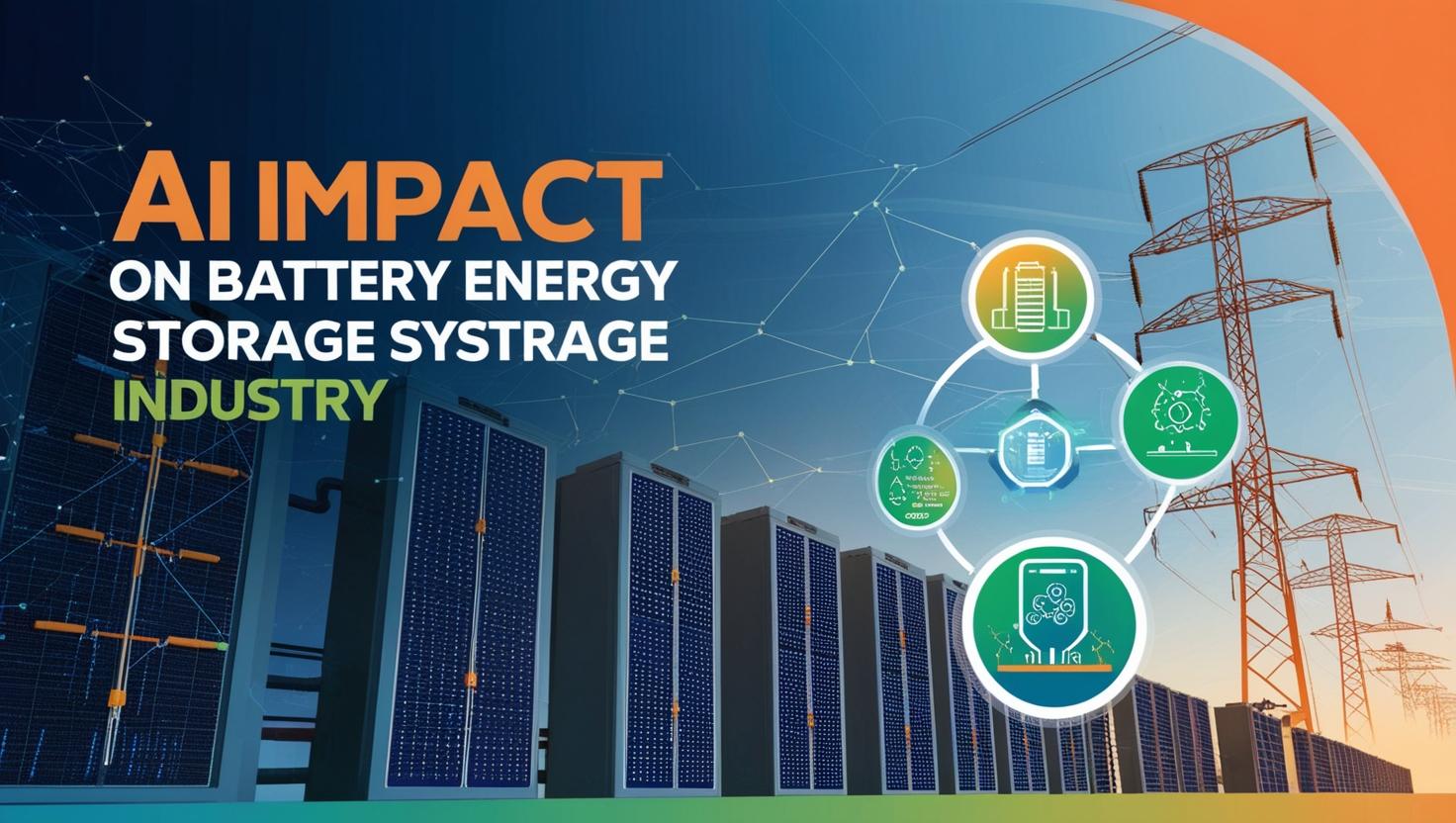
AI in Battery Research and Development: Accelerating Innovation
Beyond operational enhancements, the AI impact on battery energy storage system industry is revolutionizing research and development (R&D). Developing new battery materials and technologies is a slow and costly process, often relying on trial-and-error methods. AI accelerates this by using machine learning to simulate and predict the performance of novel materials, reducing the time and cost of experimentation.
For instance, AI models can analyze the chemical and electrochemical properties of battery materials to identify candidates with higher energy density and lower costs. This has led to breakthroughs in solid-state electrolytes and sodium-ion batteries, which promise greater safety and sustainability. The AI impact on battery energy storage system industry in R&D is also evident in digital twin technologies, which create virtual replicas of batteries for real-time testing and optimization. These advancements are critical for meeting the growing demand for high-performance, eco-friendly batteries in EVs and renewable energy storage.
Addressing Safety Concerns with AI-Powered Solutions
Safety is a critical concern in the BESS industry, particularly with lithium-ion batteries, which are prone to thermal runaway and fires. The AI impact on battery energy storage system industry addresses these risks through advanced monitoring and anomaly detection systems. AI algorithms continuously analyze battery data to detect early signs of overheating, short circuits, or chemical degradation, enabling preventive measures before catastrophic failures occur.
For example, companies like AGreatE use AI-embedded systems to monitor subtle changes in battery cells, predicting fire risks long before they materialize. These systems recommend replacing questionable modules during routine maintenance, ensuring unparalleled safety. The AI impact on battery energy storage system industry also includes cybersecurity, with AI protecting sensitive battery data from unauthorized access through encryption and real-time threat detection. By enhancing both physical and digital safety, AI is making BESS a more reliable and secure technology.
Sustainability and Cost Efficiency: AI’s Contribution to a Greener Future
The global push for sustainability has placed BESS at the forefront of decarbonization efforts, and the AI impact on battery energy storage system industry amplifies this role. AI optimizes energy usage, reducing waste and maximizing the use of renewable sources. By extending battery lifespan and improving efficiency, AI also reduces the need for frequent replacements, lowering the environmental impact of battery production and disposal.
Additionally, AI-driven cost efficiencies make BESS more accessible. Predictive maintenance and optimized energy management reduce operational costs, while AI in R&D lowers the cost of developing next-generation batteries. The AI impact on battery energy storage system industry thus aligns with environmental and economic goals, enabling a transition to a cleaner, more affordable energy future.
Challenges in Implementing AI in Battery Energy Storage Systems
Despite its transformative potential, the AI impact on battery energy storage system industry faces challenges that must be addressed for widespread adoption. One major hurdle is the complexity of integrating AI into existing BESS infrastructure. Legacy systems may lack the sensors or data architecture needed for AI applications, requiring costly upgrades. Additionally, the high computational demands of AI models, such as deep learning, can strain resources, particularly in smaller-scale BESS deployments.
Data quality and availability are also critical issues. AI relies on large, high-quality datasets to deliver accurate predictions, but many BESS operators lack comprehensive data collection systems. Furthermore, the AI impact on battery energy storage system industry must navigate regulatory and ethical considerations, such as ensuring data privacy and addressing biases in AI algorithms. Overcoming these challenges requires collaboration between industry stakeholders, policymakers, and technology providers to create standardized frameworks and scalable solutions.
Future Trends: The Evolving Role of AI in Battery Energy Storage Systems
The AI impact on battery energy storage system industry is poised to grow as technology advances and adoption accelerates. Emerging trends include the use of generative AI to design innovative battery architectures and the integration of AI with blockchain for secure, transparent energy trading in microgrids. Quantum computing, combined with AI, could further enhance battery material discovery by simulating complex chemical interactions at unprecedented speeds.
Another promising development is the rise of AI-powered smart charging systems for EVs, which optimize charging schedules based on grid capacity and renewable availability. This not only reduces grid strain but also enhances the synergy between EVs and BESS. The AI impact on battery energy storage system industry will also benefit from advancements in edge computing, enabling real-time AI processing in remote or resource-constrained environments. These trends underscore AI’s potential to drive continuous innovation in the BESS sector.
The Global Impact of AI on Battery Energy Storage System Industry
The AI impact on battery energy storage system industry is not confined to specific regions but is a global phenomenon. In Australia, energy storage capacity exceeded 1GWh for the first time, driven by AI-optimized large-scale projects. Developing nations, such as India, are leveraging AI to improve energy access through affordable, efficient BESS deployments.
International collaborations, such as those between universities and industry leaders, are accelerating AI innovation in BESS. For example, Stanford researchers have developed AI algorithms that predict battery lifespan with 95% accuracy, setting a benchmark for global R&D efforts. The AI impact on battery energy storage system industry is thus fostering a connected, sustainable energy ecosystem that transcends borders and drives collective progress toward net-zero goals.
Embracing AI for a Sustainable Energy Future
The AI impact on battery energy storage system industry is a game-changer, offering solutions to some of the most pressing challenges in energy storage and management. From enhancing battery performance and safety to optimizing grid operations and accelerating R&D, AI is unlocking new possibilities for a cleaner, more efficient energy landscape. While challenges remain, the ongoing advancements in AI technology and industry collaboration are paving the way for widespread adoption.
As the world strives for sustainability and energy security, the AI impact on battery energy storage system industry will continue to play a central role. By embracing AI-driven innovations, stakeholders can build a future where energy storage is smarter, safer, and more sustainable, powering the global transition to renewable energy with unprecedented efficiency.
FAQs
What is the AI impact on battery energy storage system industry?
The AI impact on battery energy storage system industry involves using artificial intelligence to optimize battery performance, enhance energy management, improve safety, and accelerate research and development, leading to more efficient and sustainable energy storage solutions.
How does AI improve battery lifespan in BESS?
AI improves battery lifespan by analyzing usage patterns, optimizing charge-discharge cycles, and implementing predictive maintenance to prevent degradation, ensuring batteries operate efficiently for longer periods.
What role does AI play in renewable energy integration?
AI optimizes energy storage and release in BESS, forecasting demand and supply to balance the intermittent nature of renewable sources like solar and wind, enhancing grid stability and efficiency.
What are the challenges of implementing AI in BESS?
Challenges include integrating AI with legacy systems, ensuring data quality, managing high computational demands, and addressing regulatory and ethical concerns like data privacy.
What are future trends in AI for the BESS industry?
Future trends include generative AI for battery design, AI-blockchain integration for energy trading, quantum computing for material discovery, and smart charging systems for EVs, all enhancing the AI impact on battery energy storage system industry.